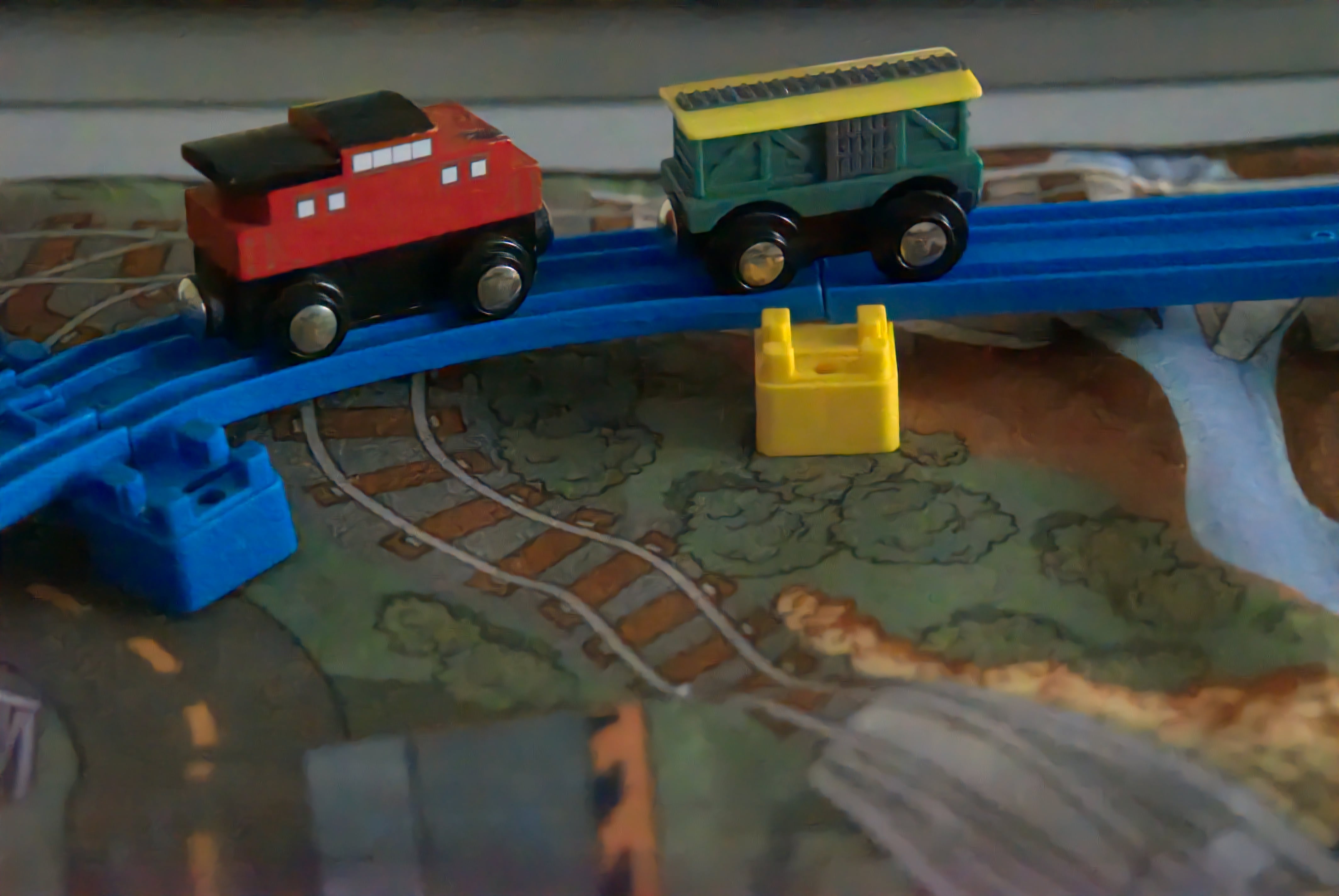
Dark Image Enhancement: DSFDNet
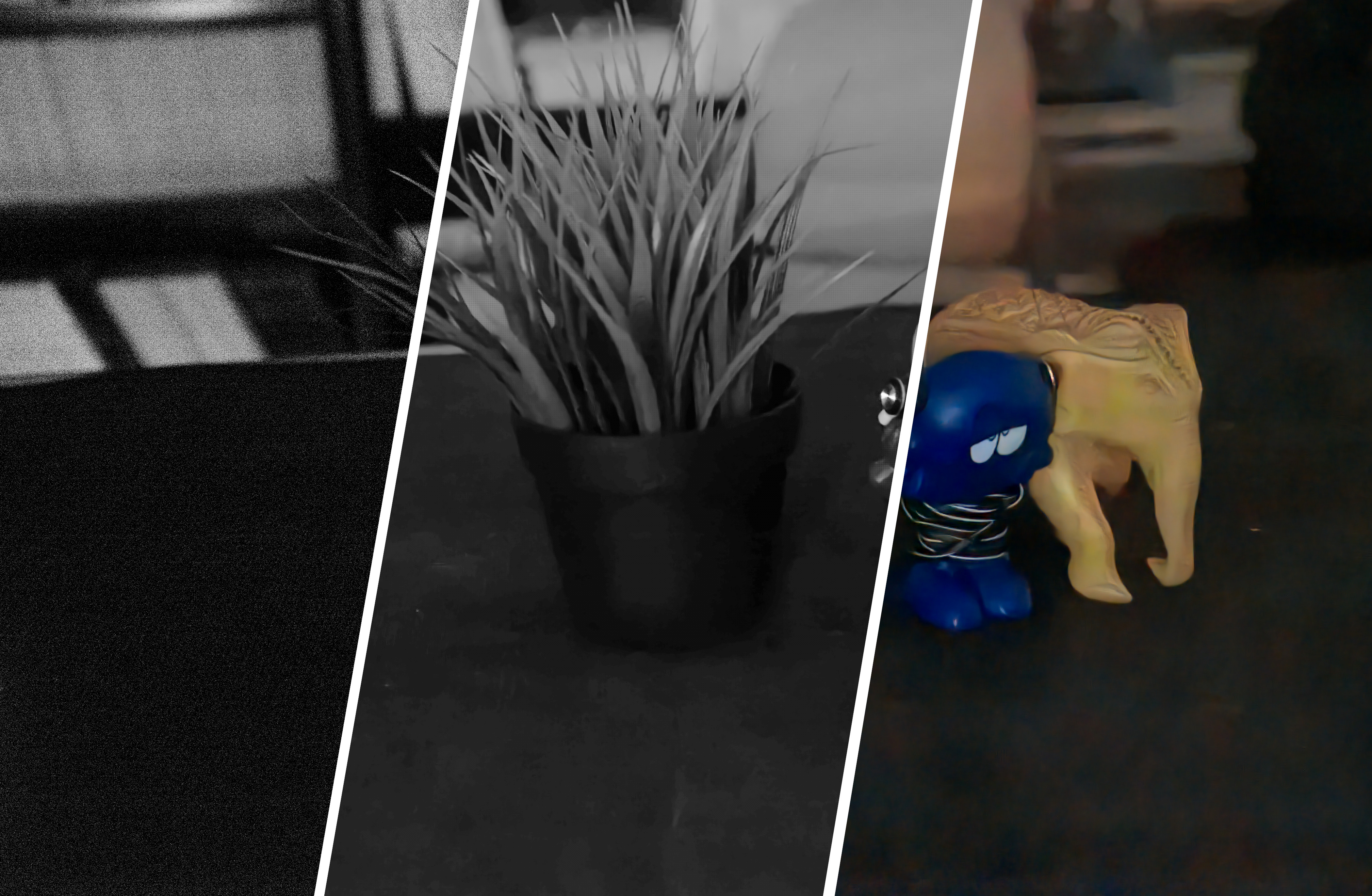
Abstract
Dark image enhancement has many interesting and valuable applications. It is a challenging problem because noises are magnified when under-exposed images are scaled up in intensity. How to better reveal the structure of the noise is the key to more effective residual learning. In this work, we developed a direct sensor field denoising solution, that takes Bayer images as input and outputs denoised Bayer images, which can be used to generate output sRGB image using off-the-shelf ISP. This not only avoids the mixing and magnifying the noises from the ISP processing pipeline, but also mitigates the need for modeling the ISP pipeline using neural networks. We further utilize wavelet decomposition to divide and conquer the problem by learning sensor field subimages with separate networks. Subimages also reveal their strong prior in noise patterns and underlying texture structure, which allow for loss function adaptation and optimization. The overall framework works directly with the sensor and can be plugged into any custom ISP pipeline. The simulation results with the See in Dark (SID) dataset demonstrate that the proposed solution achieves state of the art performance in resulting image qualities, and the direct sensor field denoising allows for further customization/optimization and performance gains in the subsequent ISP pipeline operations.
Link
Paper will be available later. Code can be found in the Github. [Code]