
Improving Extreme Low-Light Image Denoising via Residual Learning
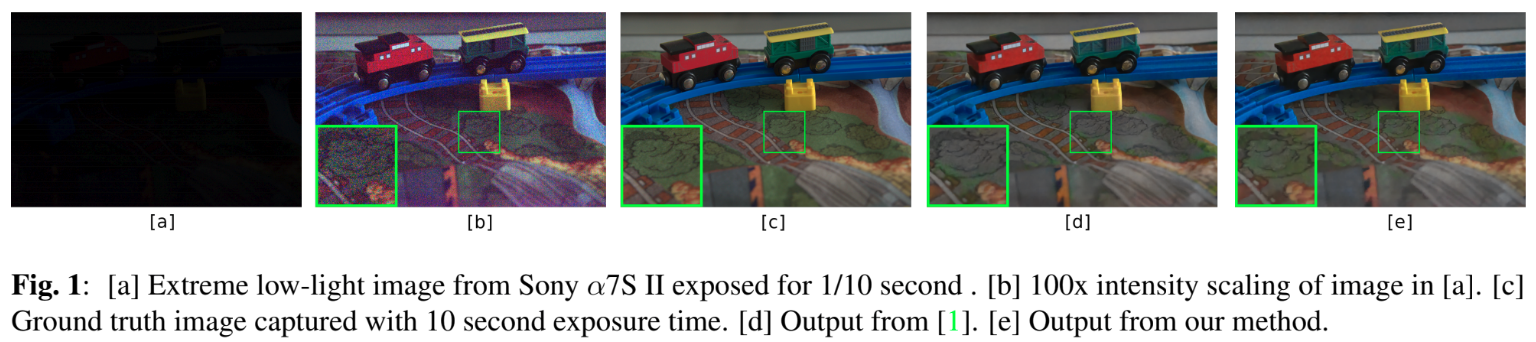
Abstract
Taking a satisfactory picture in a low-light environment remains a challenging problem. Low-light imaging mainly suffers from noise due to the low signal-to-noise ratio. Many methods have been proposed for the task of image denoising, but they fail to work with the noise under extremely low light conditions. Recently, deep learning based approaches have been presented that have higher objective quality than traditional methods, but they usually have high computation cost which makes them impractical to use in real time applica- tions or where the computational resource is limited. In this paper, we propose a new residual learning based deep neural network for end-to-end extreme low-light image denoising that can not only significantly reduce the computational cost but also improve the performance over existing methods in both objective and subjective metrics. Specifically, in one setting we achieved 29x speedup with higher PSNR. Subjectively, our method provides better color reproduction and preserves more detailed texture information compared to state of the art methods.
Link
The pdf version of the paper can be found here. [pdf]